MIT living AI Risk Repository – updates published
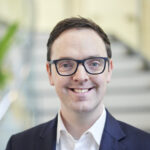
This website will offer limited functionality in this browser. We only support the recent versions of major browsers like Chrome, Firefox, Safari, and Edge.
MIT has updated its living AI Risk Repository that was launched in 2024. We summarised the key points about the repository here.
Updates include:
- Integration of 22 new AI risk frameworks, bringing the total number of included documents to 65.
- Expansion of the AI Risk Database to 1,612 unique risk entries, each systematically extracted and coded.
- Introduction of a new risk subdomain on multi-agent risks, reflecting recent developments in AI research on complex agent interactions.
According to MIT:
The Repository provides a foundation for more coherent and coordinated approaches to AI risk management. It enables:
- Risk identification and prioritization
- Development of auditing frameworks
- Improved transparency in policy and governance processes
- Identification of underexplored areas in AI safety research
The updated statistics include the following, showing that risks can be caused by various entities, with different intentions (if any), and with different timings:
Category | Level | Description | Proportion of risks in AI risk database |
Entity | Human | The risk is caused by a decision or action made by humans | 39% |
AI | The risk is caused by a decision or action made by an AI system | 41% | |
Other | The risk is caused by some other reason or is ambiguous | 20% | |
Intent | Intentional | The risk occurs due to an expected outcome from pursuing a goal | 34% |
Unintentional | The risk occurs due to an unexpected outcome from pursuing a goal | 35% | |
Other | The risk is presented as occurring without clearly specifying the intentionality | 31% | |
Timing | Pre-deployment | The risk occurs before the AI is deployed | 13% |
Post-deployment | The risk occurs after the AI model has been trained and deployed | 62% | |
Other | The risk is presented without a clearly specified time of occurrence | 25% |
When the overlap between these categories is mapped, it shows that the risks are referred to most frequently (based on how the risks have been classified rather than necessarily are present or materialize) where they are post-deployment and either 1) caused by humans intentionally, or 2) caused by AI unintentionally.
Intent | ||||
Timing | Entity | Intentional | Unintentional | Other |
Pre-deployment | Human | 2% | 4% | 1% |
AI | 1% | 2% | 1% | |
Other | - | 1% | 1% | |
Post-deployment | Human | 18% | 5% | 3% |
AI | 5% | 15% | 9% | |
Other | 2% | 2% | 4% | |
Other | Human | 3% | 2% | 2% |
AI | 3% | 4% | 2% | |
Other | 0% | 2% | 7% |
If you would like to discuss how current or future regulations impact what you do with AI, please contact Tom Whittaker, Brian Wong, Lucy Pegler, or Martin Cook. For the latest on AI law and regulation, see our blog and newsletter.